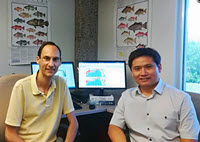
Authorities closed large portions of the Gulf of Mexico following Deepwater Horizon to minimize oil contamination of fish and seafood products. Changes in commercial and recreational fisher behavior during the closure may have caused biases in the 2010 fisheries data used to assess fish populations and establish annual quotas and catch limits.
Xuetao Lu is developing a novel modeling approach that uses statistics and computer science techniques to predict the spatial distribution of fish species. His work is part of a larger effort to expand an existing West Florida Shelf simulation model to include more fish species and fishing fleets and increase its simulated range across the Gulf. The expanded model will help researchers predict the spatial patterns of fleets and marine species under various scenarios, including oil spill events.
Xuetao is a Ph.D. student with the Arizona State University Tempe’s Statistics program and a GoMRI Scholar with the project Avoiding Surprises: Understanding the Impact of the Deepwater Horizon Oil Spill on the Decision-Making Behaviors of Fishers and How This Affects the Assessment and Management of Commercially Important Fish Species in the Gulf of Mexico Using an Agent-Based Model.
His Path
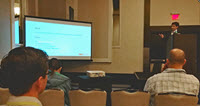
Xuetao’s favorite pastime as a teenager was playing maze games, searching for the best route as well as the correct one. While working towards his systems engineering undergraduate degree at the National University of Defense Technology in China, he realized that his fascination with mazes stemmed from a passion for understanding complex systems. “I’m fascinated by the beauty of statistics, which is the origin of many methodologies for working with complex systems,” he said. “My strong sense of curiosity led me to pursue a doctoral degree in statistics.”
Later, Xuetao was searching for graduate research opportunities, and a friend recommended that he look into Dr. Steven Saul’s research investigating quantitative approaches to Gulf of Mexico natural resource management. The team’s focus on how fisheries closures and oil pollution may have affected resource management following Deepwater Horizon excited Xuetao and made him eager to see his statistical research inform policy development and resource management decision-making. He applied for a doctoral research position in Dr. Saul’s lab and joined his team in 2017.
His Work
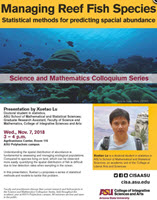
Xuetao’s ultimate goal is to develop spatial distributions of fish abundance by species, which the team will use in their model simulations of fish abundance and biomass locations. His approach utilizes bottom longline survey data (for deep water species) and video survey data (for shallow water species) collected by the National Marine Fisheries Service (NMFS). NMFS conducts independent video surveys each year to measure fish abundance; however, low detection rates generate data that is zero inflated, meaning that zero or near-zero fish appear in each sample. As a result, it is difficult for researchers calculating spatial distribution to utilize this valuable data.
Xuetao addressed this challenge by developing statistical models based on empirical maximum likelihood analysis, a technique that estimates how many fish live in an area despite low detection rates. Then, he developed a random smoothing method that uses variance and credibility factors to identify and eliminate uncertainty within the data and generate high-quality data without high uncertainty. The random smoothing method also converted the maximum estimate number of fish into the maximum estimate density of fish, which researchers can use to determine spatial distribution.
Xuetao combined the improved data with habitat information (such as depth, sediment type, or rugosity) gathered from oil company surveys so that his model could determine how different habitat features affect fish’s spatial distribution and how this relationship can predict spatial distribution in unsampled areas. The model utilized and integrated the results of thirty-three machine learning models designed to handle non-linear problems such as the relationship between habitat and spatial distribution. Finally, Xuetao ran his results through a hierarchical Bayesian model combined with the Gaussian process to correct a prediction bias that did not account for pollution and overfishing.
Comparing traditional linear model results and non-linear model predictions, Xuetao found that his non-linear model provided a more accurate and reasonable ecological overview and offered higher-resolution patterns than traditional linear predictions. His next step is to expand his non-linear model to analyze spatial distribution over time, which will help researchers track long-term distribution changes.
His Learning
Xuetao views Dr. Saul as a role model and mentor who taught him important research techniques to break down complex systems, including asking simple but meaningful questions. “Most importantly, Dr. Saul taught me how to improve my communication skills, how to collaborate with others, and how to build up my own networking,” he said. He applied these communication skills at the 2018 and 2019 Gulf of Mexico Oil Spill and Ecosystem Science conferences, where he presented his research. “I appreciate these opportunities to engage and communicate with scientists from the GoMRI science community,” he said. “The most exciting moments were when I got feedback and suggestions from other experienced researchers. The peer recognition inspired and encouraged me to keep walking forward.”
His Future
Xuetao looks forward to using his statistics background in a wide range of scientific and technological applications, especially as a university postdoc or faculty member. “As celebrated mathematician and statistician John W. Tukey said, the best thing about being a statistician is getting to play in everyone’s backyard. That makes being a statistician so much fun!” said Xuetao. “My advice? Interest is the best teacher. Find the field that you are most interested in – the sooner the better!”
Praise for Xuetao
Dr. Saul praised Xuetao’s hardworking personality and ability to work independently or in a group. He highlighted Xuetao’s communication skills, particularly his clear delivery and ability to distill complex information to an understandable level for various audiences and his intelligent and creative approaches to the team’s research. “Xuetao is able to independently distill a difficult quantitative problem down into its components and creatively apply statistical theory to solve the problem,” said Dr. Saul. “His innovative contributions and deep knowledge of mathematical and statistical theory play a critical role in the success of our project.” He emphasized that Xuetao’s methodologies represent important contributions toward a novel approach for understanding and computing the spatiotemporal abundance of living marine resources. “Xuetao is an emerging early career mathematician and statistician, who will be successful in whichever endeavor he pursues. I very much look forward to continued collaborations with him,” concluded Dr. Saul.
The GoMRI community embraces bright and dedicated students like Xuetao Lu and their important contributions. The GoMRI Scholars Program recognizes graduate students whose work focuses on GoMRI-funded projects and builds community for the next generation of ocean science professionals. Visit the [consortia website] to learn more about their work.
By Stephanie Ellis and Nilde Maggie Dannreuther. Contact sellis@ngi.msstate.edu for questions or comments.
************
The Gulf of Mexico Research Initiative (GoMRI) is a 10-year independent research program established to study the effect, and the potential associated impact, of hydrocarbon releases on the environment and public health, as well as to develop improved spill mitigation, oil detection, characterization and remediation technologies. An independent and academic 20-member Research Board makes the funding and research direction decisions to ensure the intellectual quality, effectiveness and academic independence of the GoMRI research. All research data, findings and publications will be made publicly available. The program was established through a $500 million financial commitment from BP. For more information, visit https://gulfresearchinitiative.org/.
© Copyright 2010-2019 Gulf of Mexico Research Initiative (GoMRI) – All Rights Reserved. Redistribution is encouraged with acknowledgement to the Gulf of Mexico Research Initiative (GoMRI). Please credit images and/or videos as done in each article. Questions? Contact web-content editor Nilde “Maggie” Dannreuther, Northern Gulf Institute, Mississippi State University (maggied@ngi.msstate.edu).