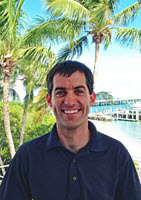
Our knowledge about ocean transport comes primarily from ocean circulation models that use field observations and theoretical motion equations to simulate ocean dynamics. Ocean models can depict large-scale circulation features accurately, but resolutions high enough to capture all scales of motion entail significant computational time and cost and are challenging or even impossible for most modern supercomputers.
Matt Grossi is developing an alternative approach that uses an artificial neural network algorithm, a type of artificial intelligence, to predict ocean transport based on information it automatically learns from field observations. This type of machine learning is considerably less computationally expensive than conventional circulation models, and Matt believes the network’s ability to digest data for skilled ocean forecasts will have many real-world applications, such as predicting oil dispersion in specific locations.
Matt is a meteorology and physical oceanography Ph.D. student with the University of Miami’s Rosenstiel School of Marine and Atmospheric Science and a GoMRI Scholar with Consortium for Advanced Research on Transport of Hydrocarbons in the Environment III (CARTHE-III).
His Path
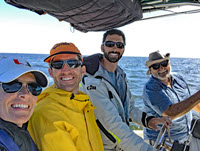
Matt credits his physical oceanography path to an eighth-grade field trip to Cape Cod, Massachusetts, where his class spent four days learning about the Cape’s geology, fauna, flora, and maritime history. A trip activity asked students to measure the speed and direction of the Cape Cod Canal surface current using a tape measure, a stopwatch, and oranges. “We hadn’t grown up near the ocean, so we had no idea that the relentless spring wind ripping through the canal could make the water appear to flow in the opposite direction of the strong tidal current,” said Matt. “Imagine how surprised we were when we tossed our oranges into the water, waited for them to float past our stopwatch, and observed them floating in the ‘wrong’ direction!”
The experience inspired Matt to pursue an undergraduate degree in physical oceanography and meteorology at the Florida Institute of Technology and then a master’s degree at the University of Delaware’s Ocean Exploration, Remote Sensing, and Biogeography lab. The Deepwater Horizon oil spill occurred while he was finishing his master’s thesis, and his lab provided targeted regional satellite products and glider resources to aid response efforts. He recalls his advisor uploading the latest satellite imagery into their models and remarking that recovery from the spill would take years – he was right. Roughly a decade later, Matt is continuing his education studying the same disaster.
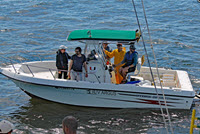
After his master’s research, Matt operated regional ocean observation systems at the University of Massachusetts Dartmouth’s School for Marine Science and Technology. Hoping to return to data exploration and research, Matt learned about Dr. Tamay Özgökmen’s GoMRI-funded ocean transport research during a recruitment visit to the University of Miami. Özgökmen described the unprecedented ocean circulation data his team had collected that was waiting to be analyzed, and Matt was excited about the broad research possibilities and the opportunity to help conduct a month-long drifter campaign in the Gulf of Mexico. He joined Özgökmen’s lab as a meteorology and physical oceanography Ph.D. student. “I am excited to engage in cutting-edge research,” said Matt. “There is a growing appreciation for the importance of the world’s ocean in understanding many of the 21st Century’s greatest environmental challenges.”
His Work
Matt is exploring how an artificial neural network (ANN) can improve predictions of ocean transport using information it learns from observational data. Rather than depending on a preexisting machine learning package, he and his colleagues are designing their own network. Unlike ocean circulation models, which use field observations to establish initial conditions and then apply theoretical algorithms to predict what should happen, their network will digest and learn from data depicting what actually happens to buoyant ocean particles.
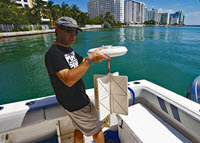
“Instead of forcing selected data into a theoretical ocean model, why not use as much field data as possible and learn what we can from it? Data-driven modeling techniques such as ANNs provide promising ways to do just that,” said Matt. “ANNs look for statistical relationships between different data sets – the more data available, the more the neural network can learn. Once trained, the network can make skilled predictions about cases not seen during training.”
The ANN’s success is dependent on (1) the data’s degree of predictability and (2) the amount of data available. Matt is currently addressing the first criteria through a proof-of-concept study assessing what information the ANN can learn about particle trajectories. He advects simulated particles in various known flow regimes, tracks their trajectories, and trains the ANN to predict where the particles will end up. So far, the group’s ANN has learned to use a particle’s previous trajectory to predict its final destination. “Our ANN’s predictions have struggled in more complicated scenarios, such as interacting scales of motion, but our model is the simplest kind of neural network and there is plenty of room for fine-tuning,” he said. “The preliminary results from these test domains have been optimistically promising, and we are now beginning similar tests using realistic oceanic flows produced by an ocean circulation model.”
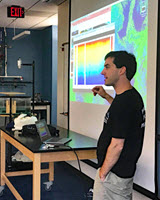
Matt’s next research step will address the second criteria concerning the amount of data available to train the ANN. While global observational ocean data are sparse, he hopes that regional observation systems and targeted field experiments will provide enough information to begin assessing machine learning’s applications for oceanography. CARTHE’s Gulf of Mexico field expeditions (the Grand Lagrangian Deployment or GLAD, the Surfzone Coastal Oil Pathways Experiment or SCOPE, the Submesoscale Processes and Lagrangian Analysis on the Shelf or SPLASH experiment, and the Lagrangian Submesoscale Experiment or LASER) represent the largest coordinated field campaigns to-date that assess interactions between mesoscale and submesoscale ocean dynamics. Matt plans to use the campaigns’ unprecedented quantities of data to assess how much oceanographic data the ANN requires to produce an accurate simulation.
While it is too early to say exactly how ocean forecasting will implement machine learning algorithms, Matt envisions a more complete picture of ocean dynamics using a network of ANNs trained for different regions and seasons. “It may sound complicated, but this is the essence of artificial intelligence: multiple machine learning algorithms working on different parts of a complex problem to achieve a common goal,” said Matt. “It’s just like people: a trained individual can only accomplish so much, but a team of trained individuals working together is always more productive.”
His Learning
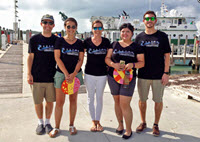
Matt said that working with Dr. Özgökmen taught him to think like a scientist and collaborate on a large research team involving multiple institutions. He was particularly grateful for his experiences working on the 2017 SPLASH experiment. “Being part of an international team of scientists working together to conduct one of the largest coordinated field campaigns to date is undoubtedly a highlight of my career,” he said. “Without the support of GoMRI, none of this would have been possible.”
His Future
Matt hopes to enter a post-doc position that will help prepare him for a research career in government, academia, or the private sector. He encourages students considering a scientific career to take advantage of any available opportunities, even if the focus isn’t related to one’s current research. He explained that opportunities to get involved are almost always available if you reach out and ask, even if they aren’t explicitly advertised. “You never know what will come of it,” said Matt. “My career started with throwing some oranges into the water in eighth grade. Many years later, I’m still throwing things into the water in the name of science, only now they’re bigger, more expensive, and have GPS tracking devices on them. I still don’t know where they’re going to go once we toss them in, but that’s what keeps things exciting – and keeps researchers employed!”
Praise for Matt
Dr. Özgökmen recruited Matt as a Ph.D. student because of his experience collecting and organizing observational data. He explained that he and Matt began considering machine learning algorithms for processing oceanic data around the same time. Matt immediately took some machine learning courses and began developing codes for processing CARTHE data, which Özgökmen expects will be instrumental to their project. Matt’s work will also help their team’s recently awarded Department of Defense Multi University Research Initiative project (with colleagues at Massachusetts Institute of Technology, University of California Los Angeles, Florida State University, and Duke University) centered on using machine learning for ocean submesoscale flows. “Submesoscale flows and machine learning for ocean data are concepts that did not really exist until the 21st Century,” said Özgökmen. “Matt is making great progress and is likely to advance oceanography in quite an exciting and different direction than usual. I hope that a lucrative career is awaiting him in the future.”
The GoMRI community embraces bright and dedicated students like Matt Grossi and their important contributions. The GoMRI Scholars Program recognizes graduate students whose work focuses on GoMRI-funded projects and builds community for the next generation of ocean science professionals. Visit the CARTHE website to learn more about their work.
By Stephanie Ellis and Nilde Maggie Dannreuther. Contact sellis@ngi.msstate.edu for questions or comments.
************
The Gulf of Mexico Research Initiative (GoMRI) is a 10-year independent research program established to study the effect, and the potential associated impact, of hydrocarbon releases on the environment and public health, as well as to develop improved spill mitigation, oil detection, characterization and remediation technologies. An independent and academic 20-member Research Board makes the funding and research direction decisions to ensure the intellectual quality, effectiveness and academic independence of the GoMRI research. All research data, findings and publications will be made publicly available. The program was established through a $500 million financial commitment from BP. For more information, visit https://gulfresearchinitiative.org/.
© Copyright 2010-2019 Gulf of Mexico Research Initiative (GoMRI) – All Rights Reserved. Redistribution is encouraged with acknowledgement to the Gulf of Mexico Research Initiative (GoMRI). Please credit images and/or videos as done in each article. Questions? Contact web-content editor Nilde “Maggie” Dannreuther, Northern Gulf Institute, Mississippi State University (maggied@ngi.msstate.edu).